Check Out Our Work!
Wildfire
Demo
Wildfire Demo Video
We present Wildfire, an innovative social sensing platform designed for laypersons. The goal is to support users in conducting social sensing tasks using Twitter data without programming and data analytics skills. Existing open-source and commercial social sensing tools only support data collection using simple keyword-based or account-based search. On the contrary, Wildfire employs a heuristic graph exploration method to selectively expand the collected tweet-account graph in order to further retrieve more task-relevant tweets and accounts. This approach allows for the collection of data to support complex social sensing tasks that cannot be met with a simple keyword search. In addition, Wildfire provides a range of analytic tools, such as text classification, topic generation, and entity recognition, which can be crucial for tasks such as trend analysis. The platform also provides a web-based user interface for creating and monitoring tasks, exploring collected data, and performing analytics.
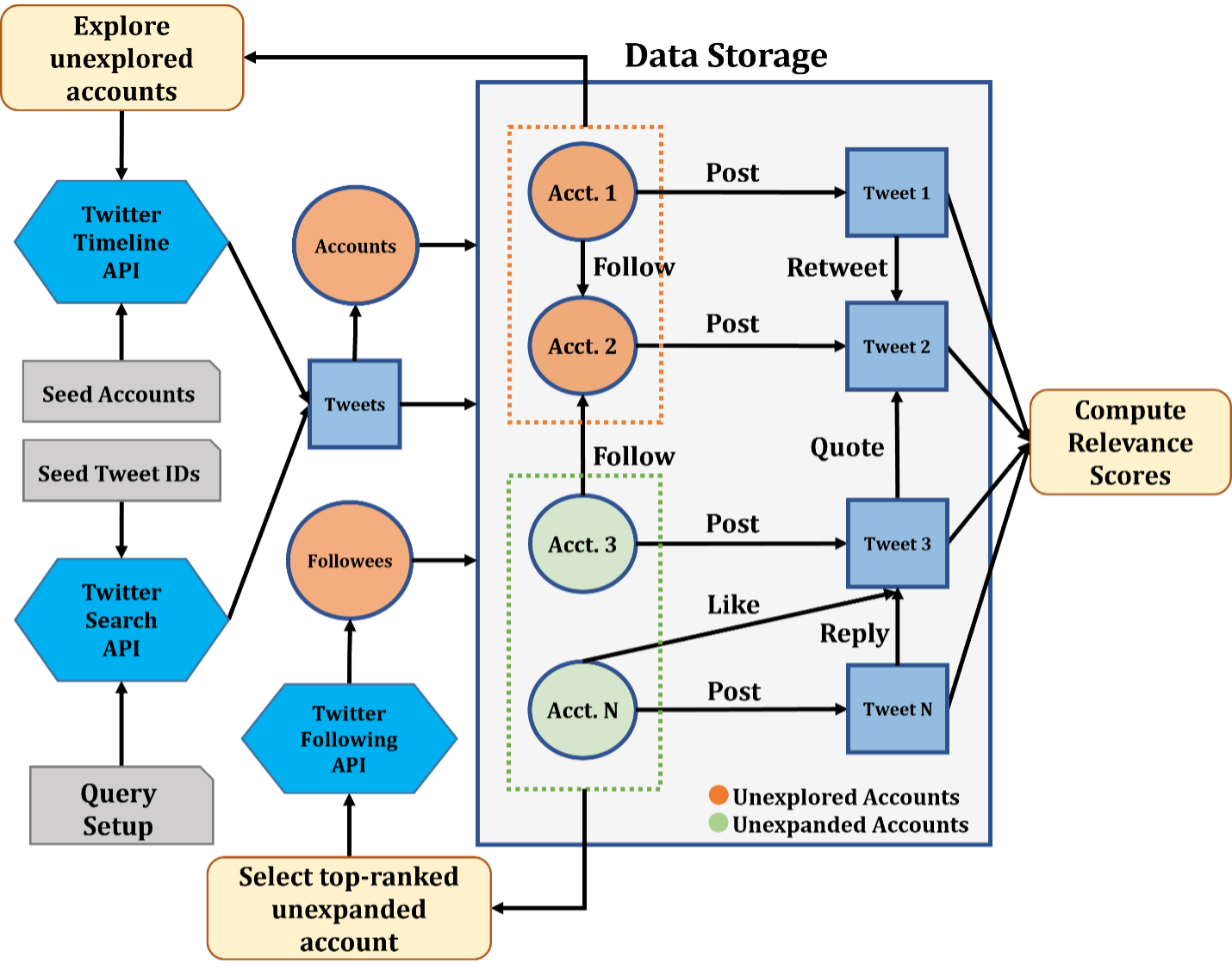
Wildfire system diagram
Exploring Behavioral Tendencies on Social Media: A Perspective Through Claim Check-Worthiness
This study examines how factual claims of different significance influence and reflect social media users' behavioral patterns. Leveraging "check-worthiness" as a measure of the factual significance of claims, we analyze the connection between factual claims and user behaviors on Twitter. Through a series of experiments using statistical methods such as correlation analysis and hypothesis testing, we provide insights into a few pivotal inquiries: (1) whether differences exist between users' tweeting tendencies toward check-worthiness, (2) the underlying reasons for such differences, (3) whether users tend to create, share, and endorse content with check-worthiness levels similar to their own tweets, and (4) whether users with similar tendencies toward check-worthiness exhibit heightened engagement. The experiments were conducted across three datasets, comprising over 48.5 million tweets and involving 15,000 users, spanning several domains and yielding statistically significant findings. Previous studies have primarily centered on examining the effectiveness and strategies of fact-checks rather than understanding people's behavioral tendencies toward factual claims. Our research pioneers understanding in this area, offering valuable insights for behavioral modeling and social sciences.
Truthfulness Stance Detection
RATSD: Retrieval Augmented Truthfulness Stance Detection
The rapid spread of misinformation on social media presents challenges for gauging the truthfulness of public discourse. This paper introduces the concept of truthfulness stance, which assesses whether a textual utterance believes a factual claim to be true, false, or expresses a neutral stance or no stance toward the claim. Our systematic analysis fills a gap in the existing literature by offering the first in-depth exploration of truthfulness stance. We propose a new framework, RATSD (Retrieval Augmented Truthfulness Stance Detection), which utilizes large language models (LLMs) with retrieved-augmented generation to enhance the contextual understanding of tweets in relation to claims. RATSD is evaluated on the newly developed TSD-CT dataset, which contains 3,105 claim-tweet pairs, alongside benchmark datasets. Our experiments show that RATSD outperforms state-of-the-art methods, achieving a significant increase in Macro-F1 score. Our contributions lay the groundwork for advancing research in misinformation analysis and provide tools for understanding public perceptions.
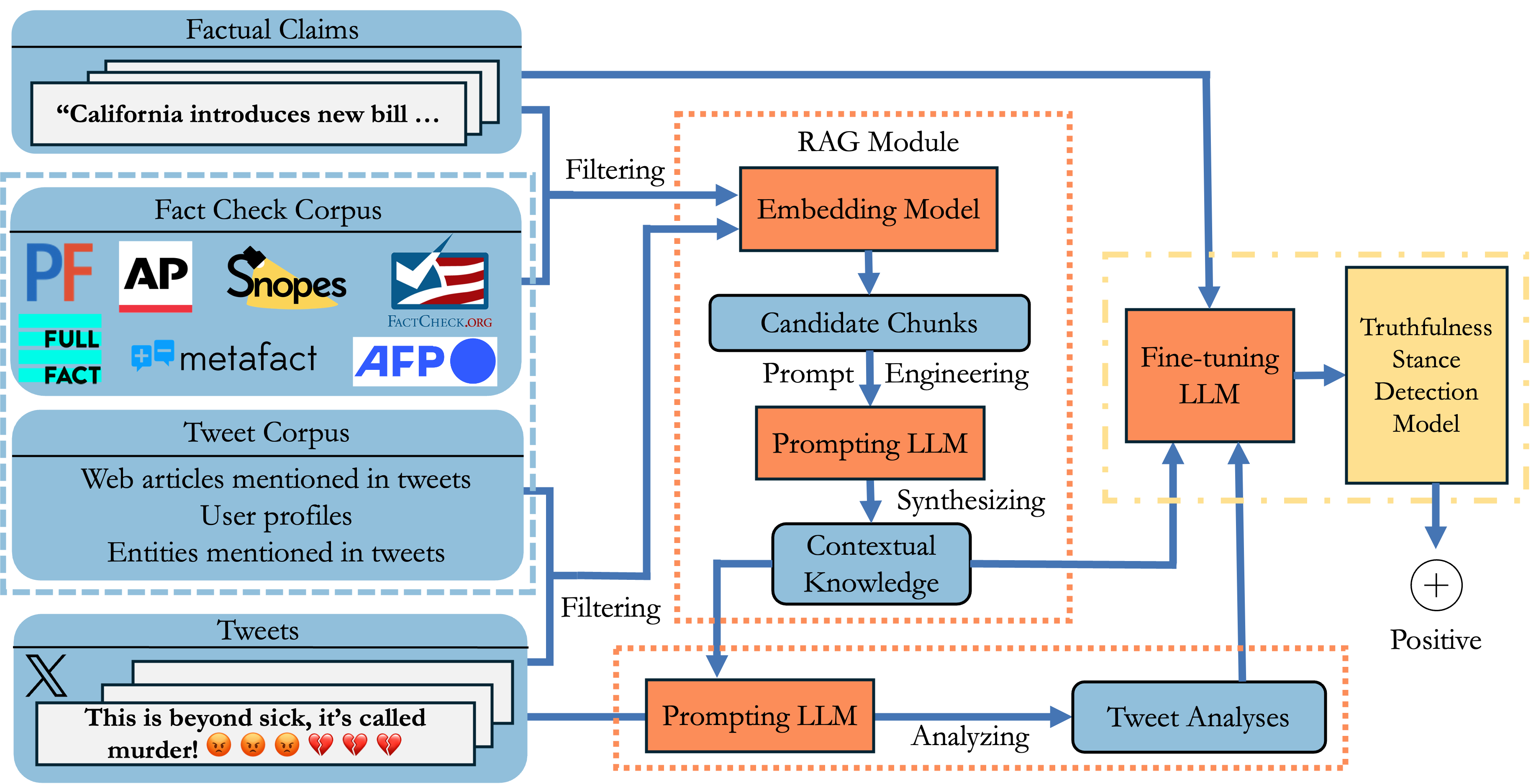
The RATSD framework
Use case: COVID-19 Dashboard
This paper describes the current milestones achieved in our ongoing project that aims to understand the surveillance of, impact of, and effective interventions against the COVID-19 misinfodemic on Twitter. Specifically, it introduces a public dashboard which, in addition to displaying case counts in an interactive map and a navigational panel, also provides some unique features not found in other places. Particularly, the dashboard uses a curated catalog of COVID-19 related facts and debunks of misinformation, and it displays the most prevalent information from the catalog among Twitter users in user-selected U.S. geographic regions. The paper explains how to use BERT-based models to match tweets with the facts and misinformation and to detect their stance towards such information. The paper also discusses the results of preliminary experiments on analyzing the spatio-temporal spread of misinformation.
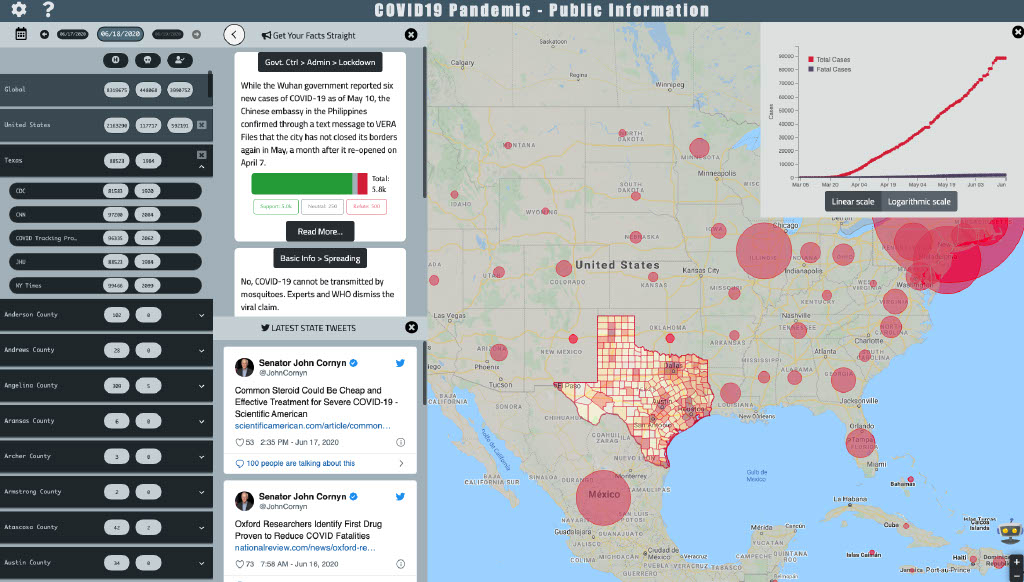