Voting Claims Fact-Checker
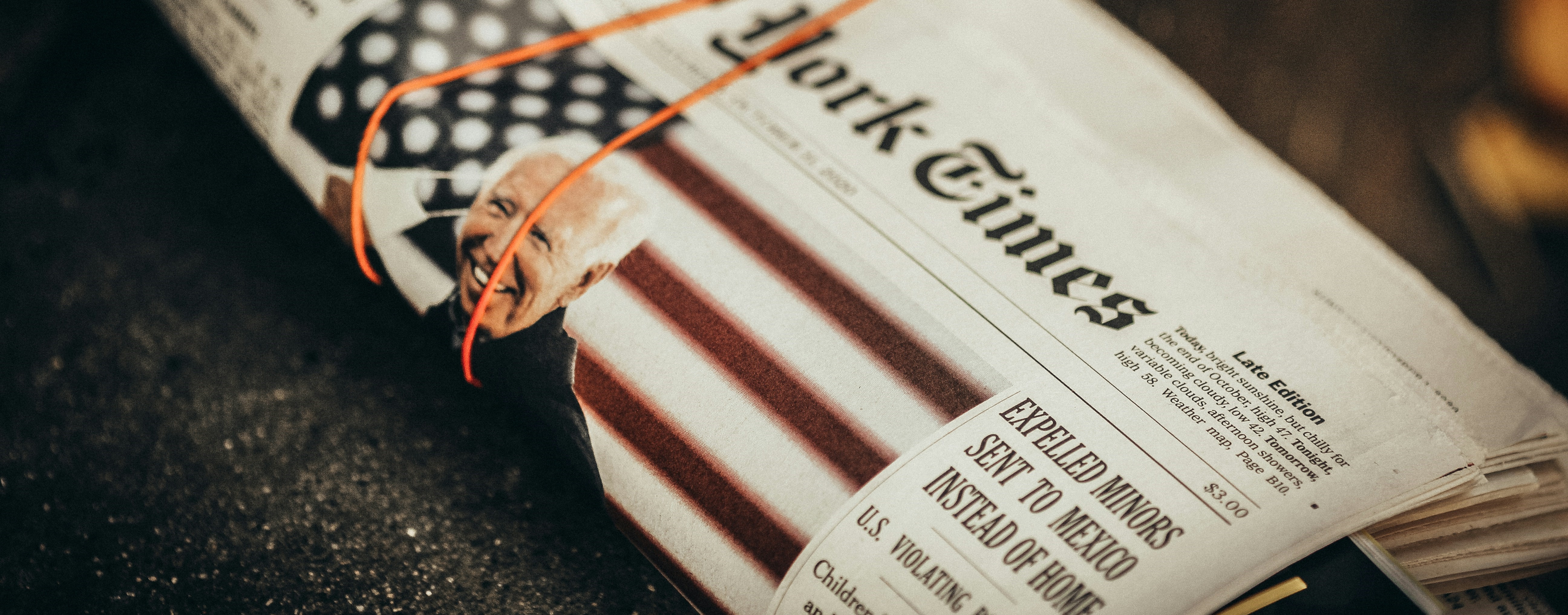
About the Project
The project aims to enhance automated fact-checking by leveraging advanced frame semantic parsing techniques to identify voting-related information. By employing state-of-the-art models, the project seeks to efficiently and accurately analyze text data for verifying the accuracy of voting-related claims and statements.
Frame Semantic Parsing
Utilizing frame semantic parsing, the project deciphers intricate sentence structures and meanings. This technique is vital for grasping contextual cues in voting-related content, enhancing accurate fact-checking.
Voting-Related Information
Focusing on election details, candidate profiles, and voting methods, the project spotlights information crucial for trustworthy fact-checking. This specialization ensures precise identification of misinformation.
State-of-the-Art Models
Employing cutting-edge models, the project leverages machine learning's pinnacle. By training on extensive datasets, these models discern intricate language patterns, fortifying accurate automated fact-checking in voting contexts.